Phase II: Call Performance Dashboards
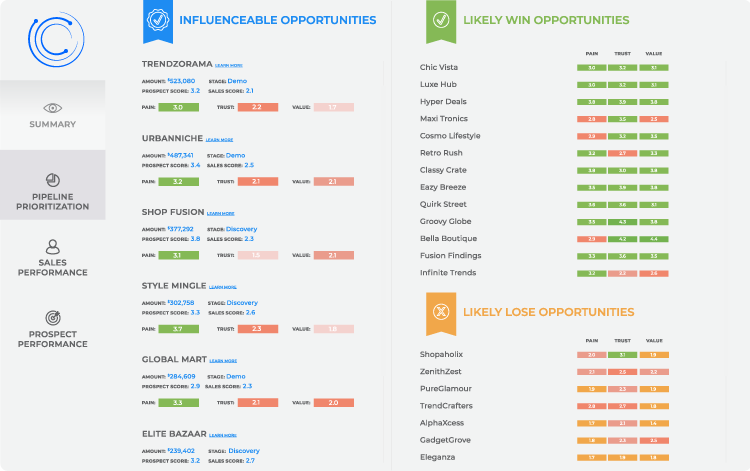
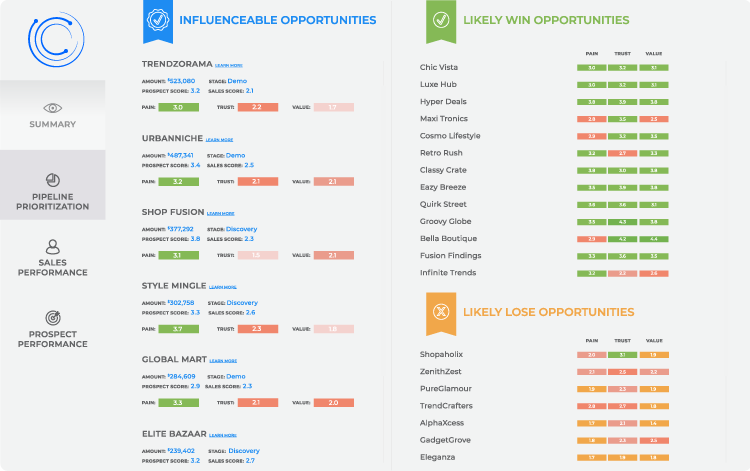
The most accurate predictive model applied incorrectly will fail to create impact.
Deploying a predictive model effectively requires an iterative process. The goal is to provide your teams a helpful yet seamless experience with the prediction engine. This involves integrating the model output within your revenue technology to both encourage positive outcomes and collect team feedback to drive model iteration.
Continuous learning and iteration is a characteristic of an effective predictive model. To ensure continuously improving accuracy, we will implement an orchestrated data loop to provide the model with new outcomes, both positive and negative, to facilitate the incremental improvement of the output. Leveraging new ecosystem data and direct team feedback, Structure Digital will continue to evolve the model output to improve revenue impact over time.
Predictive Model Integration
Outputs of the predictive model ultimately need to be available in the systems where they are most useful to internal teams. Most often, this means populating outputs into primary systems-of-truth where it benefits the teams the most.
Team Feedback Collection
Feedback loops enable sales leaders and rep end users to provide model training input through feedback about prediction accuracy. This human feedback can be used to illuminate differences between statistical analysis and personal experiences.
Continuous Monitoring
Using a predictive sales model can provide businesses with valuable insights and data-driven recommendations for optimizing their sales processes, improving sales performance, and driving revenue growth. However, it's important to continuously evaluate and refine the model to ensure its accuracy and relevance as market conditions and customer behaviors change over time. The steps to deploy a predictive model include:
Impact Measurement
We view the relationship with you as a true partnership. Winning together will take time and effort so creating real measurable results is critical to sustaining the relationship. Revenue growth is a key success measurement therefore it’s important we develop a collaborative set of success criteria to drive toward.
We were getting ghosted in the sales cycle and wanted to know why. We asked Structure Digital to get involved to figure that shit out and they nailed it. The insights they gave us helped us figure out how to adjust our process and close more deals.
